Understanding the Unpredictable Performance of Springs Health
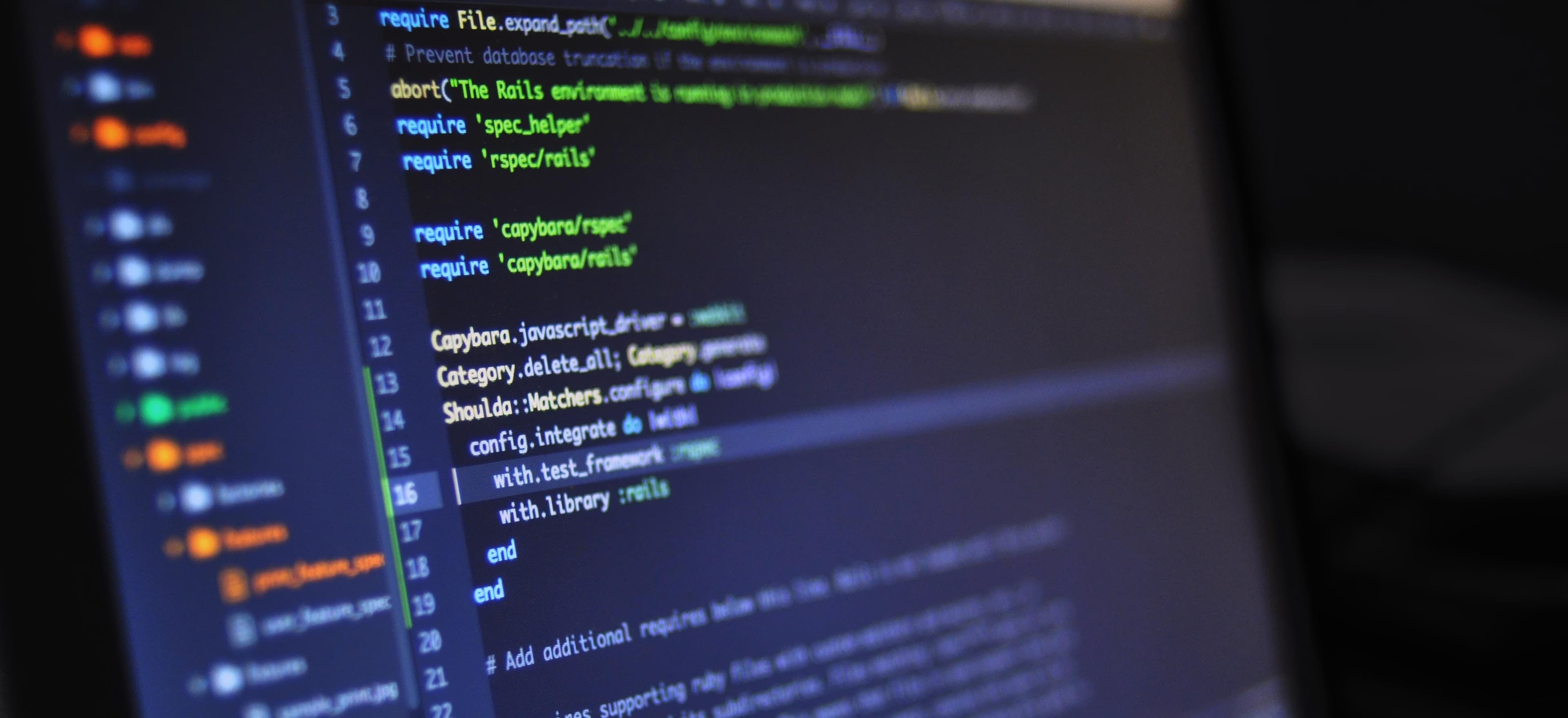
- Published on
Understanding the Unpredictable Performance of Springs Health
In today’s fast-paced world, health technology solutions are becoming increasingly crucial. Among them, Springs Health, a data-driven mental health platform, has gained attention for its innovative approach to managing mental health issues. However, many users report unpredictable performance, raising questions about its reliability and effectiveness. In this blog post, we’ll explore why this unpredictability may occur and how it impacts users.
What is Springs Health?
Springs Health utilizes advanced algorithms and data analysis to provide personalized mental healthcare solutions. By leveraging artificial intelligence (AI) and machine learning, Springs aims to create a roadmap for individuals seeking mental health support. Their approach emphasizes real-time data and user feedback to adapt and optimize mental health interventions.
The Importance of Predictable Performance
Predictable performance is essential in health care technology. Users need reliability to feel secure and confident in their care plans. Mental health, in particular, is sensitive to fluctuating support. Here are a few points showcasing the importance:
- Trust: When platforms exhibit consistency, users are more likely to trust the advice and recommendations provided.
- Engagement: Reliable platforms foster user engagement. If feedback loops are intermittent, users might be less inclined to use the application regularly.
- Outcomes: Predictability can lead to improved health outcomes. If healthcare technology consistently delivers results, users are more likely to see lasting change.
Possible Reasons for Unpredictable Performance
1. Data Variability
Health technology platforms depend heavily on data. Variability in the data can significantly affect the performance of an algorithm. Springs Health may encounter fluctuations in the quality and volume of collected data.
For instance, if users provide inconsistent feedback or do not engage frequently, the algorithm struggles to adapt in real time. This leads to a lag in personalized recommendations, causing users to feel unsupported.
2. Algorithmic Limitations
The algorithms used in Springs Health are sophisticated but not infallible. AI and machine learning models learn from historical data. If the input data is biased or not representative, the performance can suffer. For example, if the training dataset lacks diversity, the model may underperform for certain user demographics.
Further complicating matters is the black box nature of many machine learning models. Users may not see how decisions are made, leading to perceptions of unpredictability.
3. Mental Health Complexity
Mental health itself is inherently unpredictable. Factors influencing mental health can range from personal history to recent life changes. Springs Health’s algorithms may find it challenging to account for such variables, leading to inconsistencies in performance.
4. User Engagement Levels
A platform like Springs Health relies on user engagement for optimal performance. If users do not actively participate—such as by updating their moods or providing regular feedback—the app's functionality can diminish. Parts of an individualized health plan might not trigger if data is not sufficient.
Addressing the Challenges
Understanding the unpredictable performance of Springs Health doesn’t mean the platform is ineffective. There are several steps the company and its users can take to improve reliability:
Encouraging User Engagement
Users can be encouraged to engage regularly with the app. By creating reminders or offering incentives, Springs Health might boost user feedback frequency. Regular updates allow the algorithms to refine and deliver more accurate recommendations.
Ongoing Algorithm Improvements
For Springs Health, continuous improvement of their algorithms is key. By testing and validating the model on diverse datasets, the platform can enhance its responsiveness. They should also consider incorporating user-experience design principles to keep the app intuitive and engaging.
Incorporating Feedback Loops
Implementing robust feedback loops can help improve reliability. Users should have the ability to express dissatisfaction or satisfaction with the recommendations provided. This allows the algorithms to learn and adapt more effectively.
User Education
Educating users about how the platform works can mitigate feelings of unpredictability. When individuals understand the nature of the algorithm and what is required from them, consistency in use may improve.
Moving Forward with Springs Health
In essence, while Springs Health has the potential to offer high-quality, personalized mental health support, inconsistencies can hinder users’ experiences. It’s crucial for the company to address these challenges and for users to engage actively with the platform.
As technology continues to evolve, so will methods to enhance the performance of mental health solutions. For more insights into algorithm reliability and mental health technology, visit Mental Health America and Harvard Health Publishing.
The Last Word
The unpredictable performance of Springs Health poses challenges for users seeking reliable mental health support. By understanding the underlying causes, users and developers can work together to create a more effective and engaging experience. With ongoing commitment to improvement and user engagement, platforms like Springs Health can reshape how we approach mental health.
In the end, confidence in health tech solutions stems from their reliability and effectiveness. Let’s work together to foster an environment where both users and technology can thrive in mental wellness.
If you have any feedback or questions regarding Springs Health or mental health technology, feel free to leave your comments below!